Accelerating AI Deployments with the Edge to Cloud Intel AI Portfolio – Intel Chip Chat – Episode 648
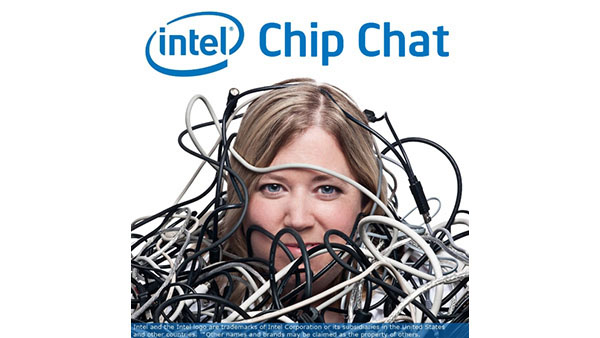
In this Intel Chip Chat audio podcast with Allyson Klein: Wei Li, Vice President of Intel Architecture, Graphics and Software, and General Manager of Machine Learning and Translation at Intel, joins Chip Chat to share Intel’s overarching strategy and vision for the future of AI and outline the company’s edge to cloud AI portfolio. Wei discusses how Intel architecture enables consistency across different platforms without having to overhaul systems. He also highlights increased inference performance with the 2nd Generation Intel Xeon Scalable processor with Intel Deep Learning Boost (Intel DL Boost) technology, introduced at Intel Data-Centric Innovation Day. Intel DL Boost speeds inference up to 14x [1] by combining what used to be done in three instructions into one instruction and also allowing lower precision (int8) across multiple frameworks such as TensorFlow, PyTorch, Caffe and Apache MXNet. He also touches on the work Intel has done on the software side with projects like the OpenVINO toolkit – which accelerates DNN workloads and optimizes deep learning solutions across various hardware platforms. Finally, Wei outlines future AI integrations in Intel Xeon Scalable processors, like support for bfloat16.
For more on Intel AI and the wide range of offerings and products, please visit:
intel.ai
[1] 2nd Generation Intel Xeon Scalable processors with Intel Deep Learning Boost provide up to 14x faster inference in comparison to 1st Generation Intel Xeon Scalable processors in July 2017, for details see:
intel.ai/2ndgenxeonscalable
Software and workloads used in performance tests may have been optimized for performance only on Intel microprocessors. Performance tests, such as SYSmark and MobileMark, are measured using specific computer systems, components, software, operations and functions. Any change to any of those factors may cause the results to vary. You should consult other information and performance tests to assist you in fully evaluating your contemplated purchases, including the performance of that product when combined with other products. For more information go to www.intel.com/benchmarks.
Performance results are based on testing or projections as of 7/11/2017 to 4/1/2019 and may not reflect all publicly available security updates. See configuration disclosure for details. No product can be absolutely secure. Results have been estimated or simulated using internal Intel analysis or architecture simulation or modeling, and provided to you for informational purposes. Any differences in your system hardware, software or configuration may affect your actual performance.
Intel’s compilers may or may not optimize to the same degree for non-Intel microprocessors for optimizations that are not unique to Intel microprocessors. These optimizations include SSE2, SSE3, and SSSE3 instruction sets and other optimizations. Intel does not guarantee the availability, functionality, or effectiveness of any optimization on microprocessors not manufactured by Intel.
Microprocessor-dependent optimizations in this product are intended for use with Intel microprocessors. Certain optimizations not specific to Intel microarchitecture are reserved for Intel microprocessors. Please refer to the applicable product User and Reference Guides for more information regarding the specific instruction sets covered by this notice (Notice Revision #20110804).
The benchmark results may need to be revised as additional testing is conducted. The results depend on the specific platform configurations and workloads utilized in the testing, and may not be applicable to any particular user’s components, computer system or workloads. The results are not necessarily representative of other benchmarks and other benchmark results may show greater or lesser impact from mitigations.
Posted in:
Artificial Intelligence, Audio Podcast, Intel, Intel Chip Chat